At Petrotech, we specialize in advanced process control systems tailored for critical process equipment, ensuring maximum redundancy and safety integrity. Our offerings include highly sophisticated Decoking Control Systems. These are designed with SIL1, 2, or 3 safety architectures and equipped with local and remote HMI display stations. All these are suitable for both safe and hazardous areas. With Petrotech, industries can benefit from real-time equipment monitoring, advanced diagnostics, and intelligent algorithms. These help in enhancing operational reliability and reducing the risk of unplanned downtime. Learn more about how Petrotech can revolutionize your process control solutions by visiting Petrotech.
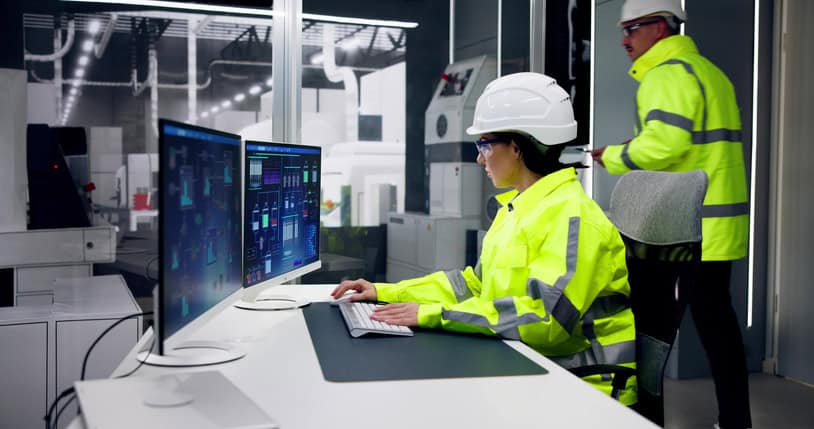
Examples of
Process Control
Process control is fundamental to the efficiency of modern manufacturing and industrial operations. This article explores various examples of process control systems, techniques for monitoring their results, and innovative alternatives to traditional methods. By understanding these elements, industries can enhance precision, efficiency, and adaptability in their processes.
Common Examples of Process Control in Industrial Settings
Process control systems are essential for managing the complex operations in industrial environments. A basic example is the thermostat, which regulates temperature automatically. Beyond this, industrial settings often utilize more complex control loops that include sensors and actuators communicating through a 4-20 mA signal to a Programmable Logic Controller or Supervisory Control and Data Acquisition system.
Programmable Logic Controller
Programmable Logic Controllers (PLCs) are specialized computers designed to replace mechanical relay sequencing with ladder logic programming. These systems are crucial for controlling a wide range of operations including high-speed processes, motor activations, mechanical actuations, robotics, and production lines. PLCs can monitor and control hundreds of devices within a plant, proving essential for both simple and complex operational tasks.
Supervisory Control and Data Acquisition
Supervisory Control and Data Acquisition (SCADA) systems gather and analyze data from various points across a manufacturing site. Thus, enabling real-time monitoring and decision-making. Essential for managing complex processes, SCADA systems work in conjunction with PLCs to enhance system visualization and monitoring. This integration boosts the effectiveness of industrial automation, ensuring rapid response to operational changes and reducing downtime.
Distributed Control Systems
Distributed Control Systems (DCS) regulate large-scale industrial operations with comprehensive control over complex processes. These scalable systems are adaptable to any plant’s specific needs and integrate multiple control layers for efficient operation. DCSs can operate independently or with PLCs, featuring configurable control algorithms and the capacity for expansion with additional programming and software. They standardize Human Machine Interface (HMI) and alarm systems, enhancing system reliability and response times for critical processes.
Techniques to Monitoring Process Control Results
Effective process control is not just about setting parameters but also about monitoring and adjusting them based on real-time data. Techniques to analyze process control results are crucial for maintaining operational efficiency and safety.
Data Visualization: Techniques like histogram plotting or real-time graphing help visualize trends and deviations in process control. Thereby making it easier to understand the system’s performance at a glance.
Statistical Process Control (SPC): Statistical Process Control (SPC) uses statistical techniques to monitor and regulate processes. This method is effective in identifying variability, which helps in implementing timely corrective actions to keep the process within designated limits and improve quality control. SPC is especially valuable in environments like manufacturing and production lines where consistency is critical.
Advanced Analytics: Advanced analytics integrates big data and machine learning algorithms into process control, enabling not just monitoring but also prediction of process behaviors. This technology can foresee future trends and potential faults, facilitating proactive adjustments to avert failures. Machine learning models play a crucial role by analyzing both historical and real-time data to identify patterns that could lead to breakdowns. Thus, allowing for preventative maintenance that ensures uninterrupted production and lowers costs.
Alternatives to Common Industrial Process Control Systems
While traditional process control examples like PID (Proportional, Integral, Derivative) controllers are widely used, there are robust alternatives. Some of these offer various advantages that are useful especially in complex or highly dynamic environments.
Fuzzy Logic
This methodology contrasts traditional process control examples like PID controllers that rely on fixed parameters and linear relationships. Instead, fuzzy logic employs a system of fuzzy sets and user-defined rules to adaptively manage control actions. This approach enables it to respond dynamically to feedback and desired outcomes. As a result, it can effectively handle nonlinearities, disturbances, and rapidly changing conditions that can confound more rigid control systems.
Fundamental to fuzzy logic are linguistic variables—terms like “low,” “medium,” and “high”—that provide a range of truth. Rather than binary true/false outputs. This nuanced approach to data interpretation allows controllers to operate on a spectrum of possibilities. Thereby making decisions that closely mimic human reasoning. Consequently, fuzzy logic is instrumental in complex and unpredictable settings such as robotics, where it improves decision-making under ambiguous conditions and enhances system adaptability.
Neural Networks
Neural networks are advanced computational models that replicate the functions of human brain neurons. Thus, enabling them to learn from large amounts of data and adapt to new scenarios. These controllers are invaluable for managing complex or nonlinear system dynamics in industrial automation, such as robotics. As they process operational data, neural networks continually refine their decision-making capabilities, thereby improving their accuracy and efficiency over time.
However, the performance of neural networks heavily depends on the quality and volume of the training data. Insufficient or low-quality dataset can significantly impair their effectiveness.
Reinforcement Learning
Reinforcement Learning (RL) is a branch of machine learning that optimizes decision-making through a trial-and-error process. This process is guided by a reward function and allows controllers to autonomously evaluate and refine their actions depending on previous outcomes. Thus, making it especially suitable for dynamic or uncertain environments.
RL is adept at enabling robots to adapt and develop autonomous behaviors that surpass the capabilities of traditional PID controllers. This facilitates advanced adaptability and problem-solving skills in complex settings. However, the effectiveness of RL depends heavily on the intricacy of the reward function. So this function must be precisely engineered to drive desired behaviors.
Model Predictive Control
Model Predictive Control (MPC) is an advanced control technique. It utilizes a mathematical model of the system and its constraints to optimize control actions over a specified time horizon. Unlike PID controllers, MPC excels in managing multivariable systems, nonlinearities, constraints, and disturbances, while also considering future scenarios and trade-offs. Despite its effectiveness, MPC demands a dependable and precise system model. In addition, it requires substantial computational power and memory resources to operate efficiently.
Sliding Mode Control
Sliding mode control employs a switching control law to guide system states towards a predefined “sliding surface,”. It does this along with effectively managing fluctuating dynamics or external disturbances. This control method excels in delivering robust and swift performance, adept at handling uncertainties and nonlinearities. However, it can produce high-frequency oscillations, known as chattering, which may impair system efficiency or integrity. Optimal performance also depends on the careful selection of the sliding surface and switching parameters.